Over the past years, I have gotten deeper and deeper into 3d-printing. One thing that fascinates me a lot is how different it is from other manufacturing methods. And how this, in turn, means a completely different design philosophy is needed to create good designs for 3d-printing.
As such, I have been collecting the little tricks and rules for designing well-printable parts. And of course I am always on the hunt for more. In this blog post I want to share all that I have learned.
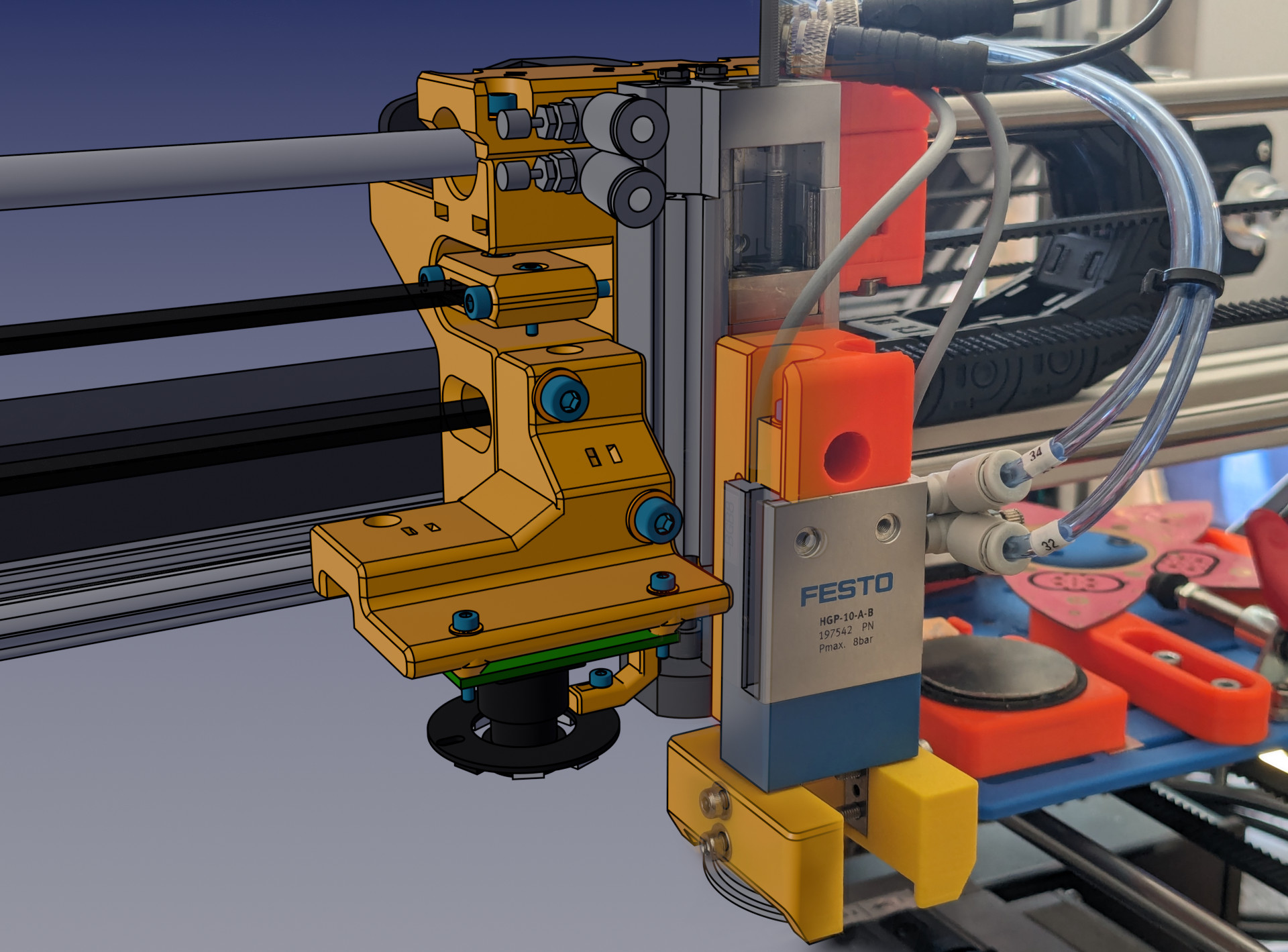